Chi-Squared Test: Skills (DP IB Biology)
Revision Note
Chi-squared Test
Looking for associations between species
The distribution of species in a habitat is rarely random; it usually depends on factors such as soil type, water availability, and competition
It is sometimes possible to observe an association between the distributions of different species within a habitat, e.g.
Species that are in a symbiotic relationship are likely to be found next to each other; we would say that there is a positive association between the distributions of these two species
Species that are in direct competition for the same resources will exclude each other from their immediate surroundings, and so are likely to be found in different parts of a habitat; there might be a negative association between the distributions of these two species
If species have no interaction with each other, then there will be no association between their distributions, and any that appears to occur will be due to chance
We would say that such species have distributions that are independent of each other
Random sampling with quadrats, along with a statistical test called the chi-squared test, can be used to test for an association between two species
Using a quadrat diagram
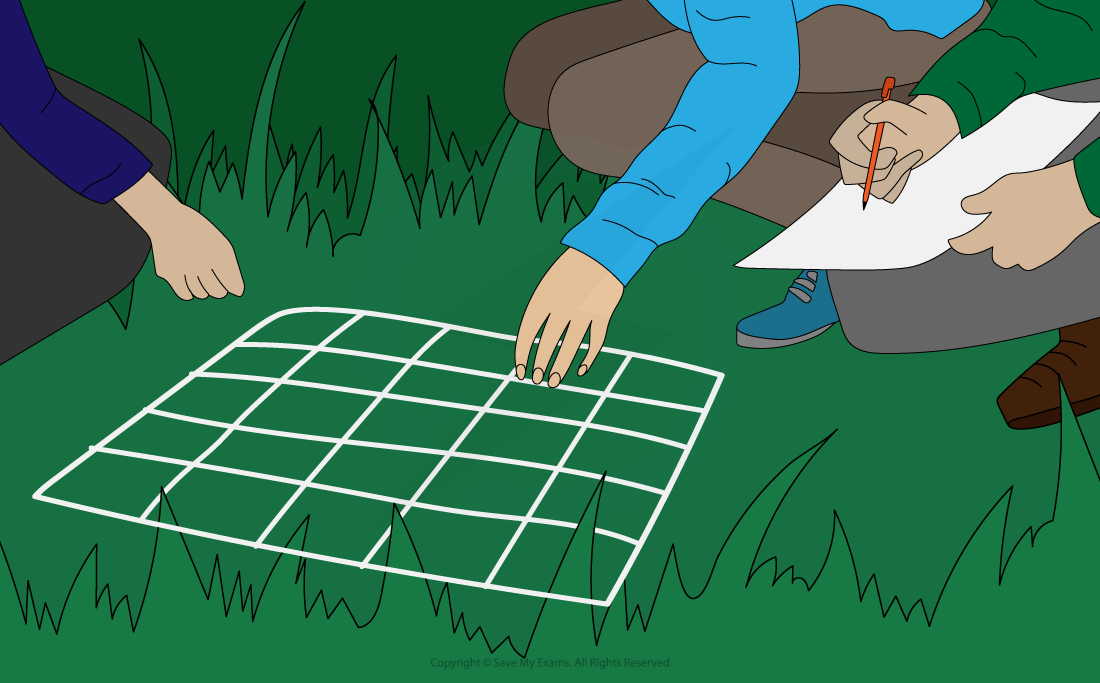
Random sampling with quadrats can be used to study the distribution of organisms
The chi-squared test
A statistical test called the chi-squared test determines whether or not there is a significant difference between the observed and expected results in an experiment
Its purpose is to assess whether any difference in these results is due to chance, or due to an association between the variables being tested
A chi-squared test can be used to analyse data from quadrat sampling to determine whether or not there is a statistically significant association between the distributions of two species
To the eye there may appear to be an association between the two species, but if it is not statistically significant then researchers can conclude that species distributions are independent of each other, and any appearance of association is due to chance
If an association is statistically significant then it must be due to an important factor, such as a symbiotic relationship
A chi-squared test enables scientists to test hypotheses
A hypothesis is a testable statement about the expected outcome of an experiment
There are two types of hypothesis:
A null hypothesis states that there is no significant difference, or association, between data sets e.g. that there is no association between the distributions of two species
An alternative hypothesis states that there is a significant difference, or association, between data sets e.g. that there is an association (either positive or negative) between the distributions of two species
The result of a chi-squared test enables scientists to either accept or reject a null hypothesis
Using the chi-squared test to test for association
Step 1: Construct a contingency table for your results
This allows the number of quadrats that contain one, both, or neither species to be recorded
Step 2: Calculate the row, column, and overall totals for your contingency table
Step 3: Calculate the expected values (E) for your table
The results recorded in the contingency table are the observed values (O); to calculate the chi-squared value we need to calculate the expected values for each data point.
The expected values are what we would expect to see if the null hypothesis were correct
Note that this is the first step towards calculating the chi-squared value, the equation for which is:

Σ = sum of O = observed value E = expected value
Step 4: Calculate the difference between the observed and expected values
Subtract the expected values from the observed values (O - E); some of the resulting values will be negative
Step 5: Square each difference
This eliminates negative values
Step 6: Divide each squared difference by the expected value
Step 7: Add all of the results from step 6 together
This gives the chi-squared value
Step 8: Calculate the degrees of freedom
Step 9: Establish a probability level or p-value
As biologists, we work with a probability level of 0.05, or 5 %
This means that we can be 95 % certain that any significant difference or association is not due to chance
Some studies require a higher level of certainty than this, e.g. medical researchers may use a smaller p-value
Step 10: Use a critical values table and the results of steps 8-9 to find the critical value
In order to understand what the chi-squared value says about the data, a table relating chi-squared values to probability is needed; this critical values table displays the probabilities that the differences between expected and observed values are due to chance
Step 11: Compare the chi-squared value with the critical value to assess the significance
Worked Example
A researcher decided to test for an association between the distribution of two types of mollusc on a rocky shore; limpets and dog whelks.
Their null hypothesis was that there was no association between the distributions of limpets and dog whelks.
They carried out 50 randomly placed quadrat samples on the rocky shore, recording either the presence or the absence of both limpets and dog whelks in each quadrat. They obtained the following results:
Quadrats containing limpets only: 14
Quadrats containing dog whelks only: 21
Quadrats containing both limpets and dog whelks: 7
Quadrats containing neither limpets nor dog whelks: 8
Use the chi-squared test to determine whether or not there is a statistically significant association between the distributions of limpets and dog whelks.
Answer:
Step 1: Construct a contingency table
Contingency table
| Limpets present | Limpets absent |
---|---|---|
Dog whelks present | 7 | 21 |
Dog whelks absent | 14 | 8 |
Step 2: Calculate the row, column, and overall totals for your contingency table
| Limpets present | Limpets absent | Row Total |
---|---|---|---|
Dog whelks present | 7 | 21 | 28 |
Dog whelks absent | 14 | 8 | 22 |
Column Total | 21 | 29 | 50 |
Step 3: Calculate the expected values
The equation for working out the expected values is:
E.g. to calculate the expected value for the category in which both dog whelks and limpets are present:
= 11.76
Step 4: Calculate the difference between the observed and expected values
O = 7
E = 11.76
7 - 11.76 = -4.76
Step 5: Square each difference
-4.76² = 22.66
Step 6: Divide each squared difference by the expected value
22.66 ÷ 11.76 = 1.93
Repeat steps 3-6 for all of the results in the contingency table:
| O | E | O-E | (O-E)2 | (O-E)2/E |
---|---|---|---|---|---|
Limpets only | 14 | 9.24 | 4.76 | 22.66 | 2.45 |
Dog whelks only | 21 | 16.24 | 4.76 | 22.66 | 1.4 |
Both dog whelks and limpets | 7 | 11.76 | -4.76 | 22.66 | 1.93 |
Neither dog whelks nor limpets | 8 | 12.76 | -4.76 | 22.66 | 1.78 |
Step 7: Add all of the results from step 6 together to obtain the chi-squared value
2.45 + 1.4 + 1.93 + 1.78 = 7.56 (this is the chi-squared value)
Step 8: Calculate the degrees of freedom
Degrees of freedom can be calculated using the following equation:
Degrees of freedom = (number of columns - 1) x (number of rows - 1)
Columns and rows refer to the original contingency table
In this example, there are 2 columns and 2 rows in the contingency table
Degrees of freedom = (2 - 1) x (2 - 1)
= 1 x 1
= 1
Step 9: Determine the probability level
As biologists, we work at a probability of 0.05, or 5%
Step 10: Use a critical values table and the results of steps 8-9 to find the critical value
Degrees of freedom | Probability that the difference between O and E is due to chance | |||
---|---|---|---|---|
0.1 | 0.05 | 0.01 | 0.001 | |
1 | 2.71 | 3.84 | 6.64 | 10.83 |
2 | 4.6 | 5.99 | 9.21 | 13.82 |
3 | 6.25 | 7.82 | 11.34 | 16.27 |
4 | 7.78 | 9.49 | 13.28 | 18.46 |
With degrees of freedom as 1, and a probability level of 0.05, the critical value can be read from the table as 3.84
Step 11: Compare the chi-squared value with the critical value to assess significance
The chi-squared value of 7.56 is larger than the critical value of 3.84
This means that there is a significant association between the two species (see section below on statistical significance)
Statistical significance
The chi-squared value, once calculated, can be compared to a critical value; this allows statistical significance to be assessed
If the chi-squared value is larger than the critical value, there is a statistically significant difference between observed and expected values, or a statistically significant association between two sets of results
In this case, the null hypothesis can be rejected
If the chi-squared value is equal to or smaller than the critical value, there is no statistically significant difference between observed and expected values, or no statistically significant association between two sets of results
In this case, the null hypothesis can be accepted
To determine the critical value biologists generally use a probability level, or p-value, of 0.05, or 5 %
This means that if a difference or association is shown to be statistically significant at this level, there is only a 5 % probability (i.e. probability = 0.05) that this result might be due to chance
Examiner Tips and Tricks
When calculating a chi-squared value it is very helpful to create a table like the one seen in the worked example. This will help you with your calculations and make sure you don’t get muddled up!
You've read 0 of your 5 free revision notes this week
Sign up now. It’s free!
Did this page help you?