Outliers, High-Leverage & Influential Points (College Board AP® Statistics)
Revision Note
Outliers in a regression model
What is an outlier in a regression model?
An outlier in a regression model is a point that has an extreme
-value relative to the least-squares regression line
It has a very large (positive or negative) residual
It does not follow the general linear trend shown by the rest of the data
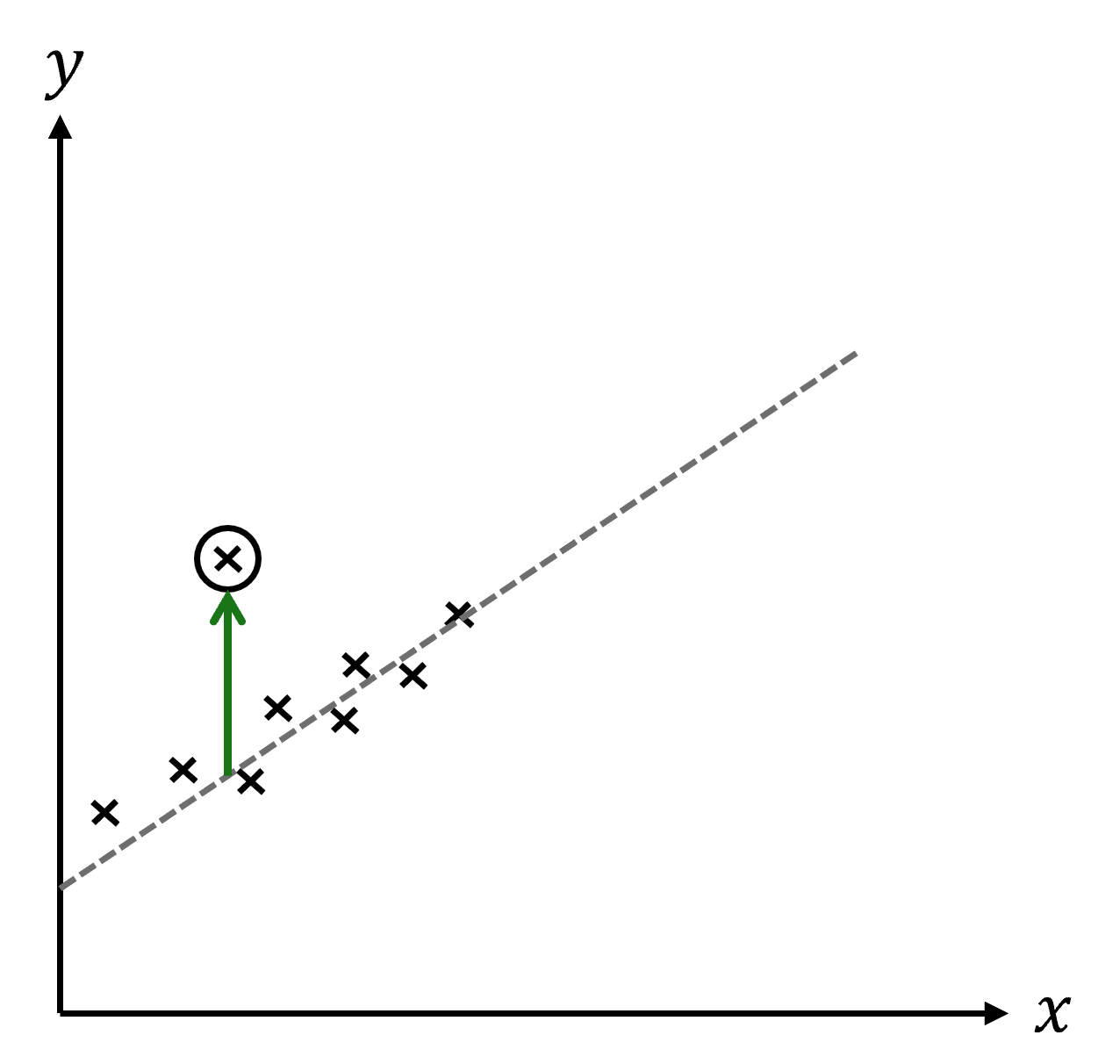
High-leverage points
What is a high-leverage point?
A high-leverage point is a data point that has an extreme
-value relative to the other data points
A high-leverage point may still follow the general linear trend shown by the data
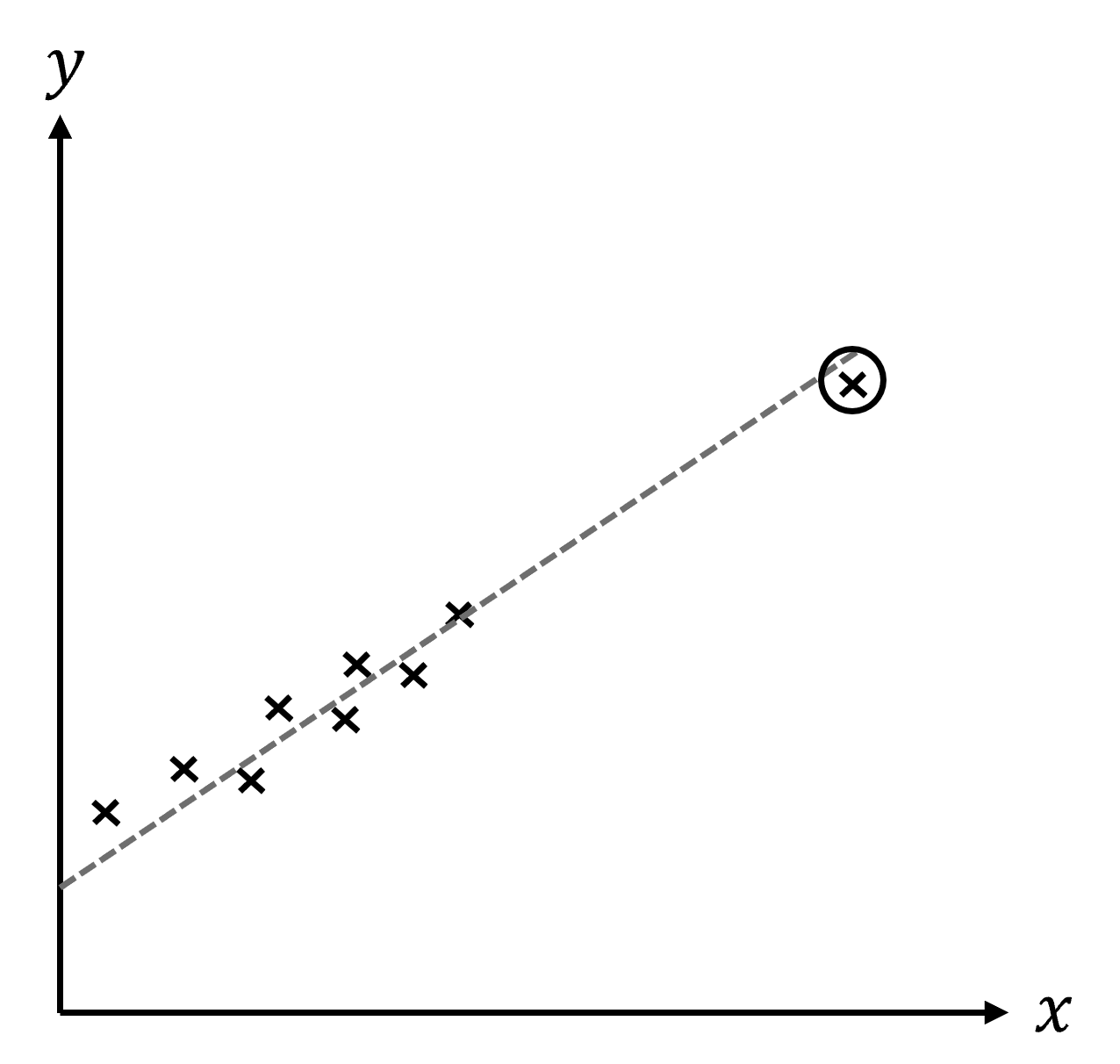
Can a high-leverage point also be an outlier?
A high-leverage point can also be an outlier if it has both:
an extreme
-value relative to the other data points
an extreme
-value (large residual) relative to the regression line
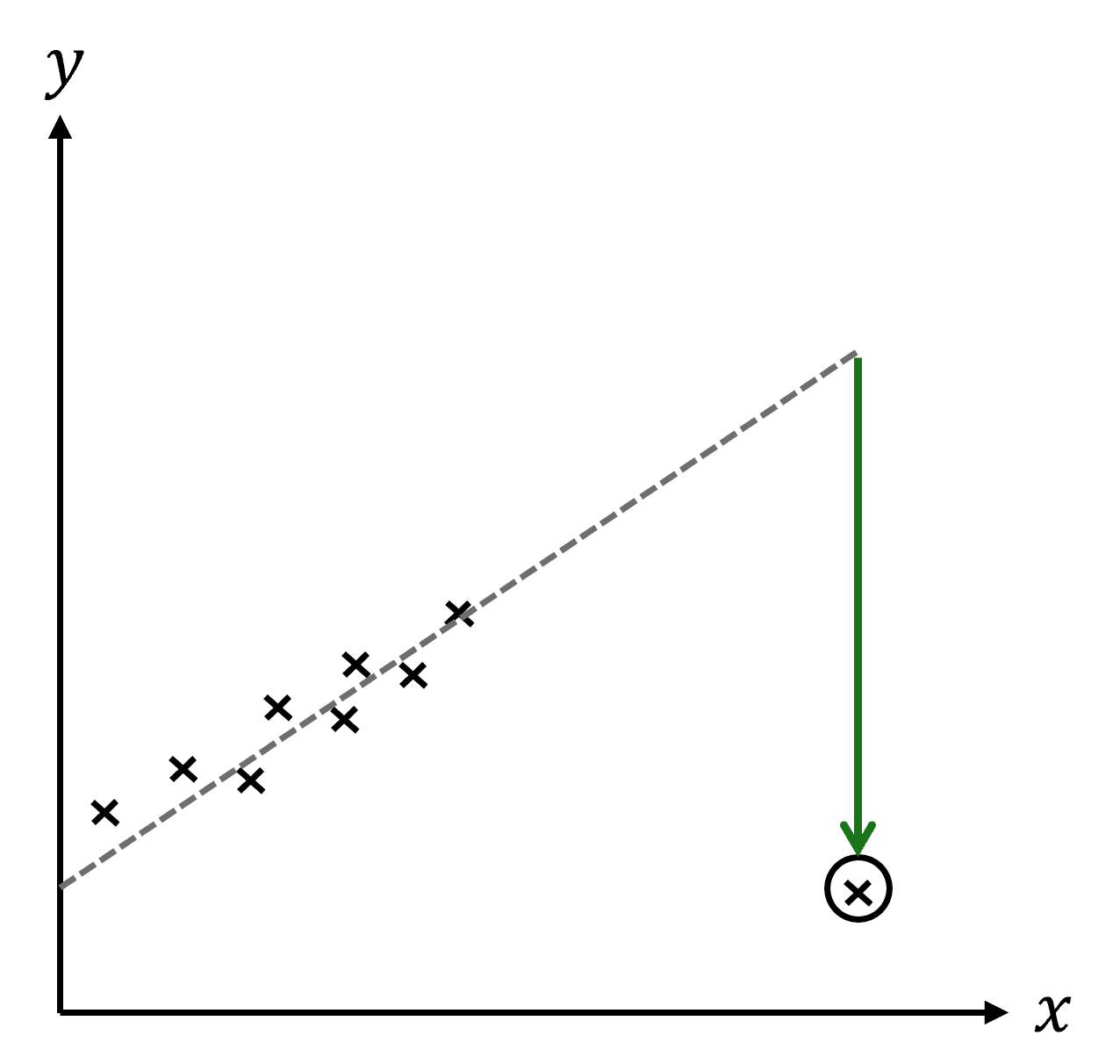
Note that a high-leverage point that does follow the general linear trend is not considered an outlier
This is because, even though it has an extreme
-value, it still lies close to the regression line
so does not have a large residual
Influential points
What is an influential point?
An influential point in a regression model is a point that, if removed, changes the linear relationship significantly
Removing it could cause a significant change in:
the correlation coefficient
the slope of a regression line
the
-intercept of a regression line
Can outliers and high-leverage points be influential points?
An outlier that is also a high-leverage point is likely to be an influential point
it has an extreme
-value relative to the other data points
it has an extreme
-value (large residual) relative to the regression line
Including this point could significantly change the slope of the regression line, for example
Outliers or high-leverage points alone may or may not be influential points
They all affect the linear relationship
but not necessarily significantly
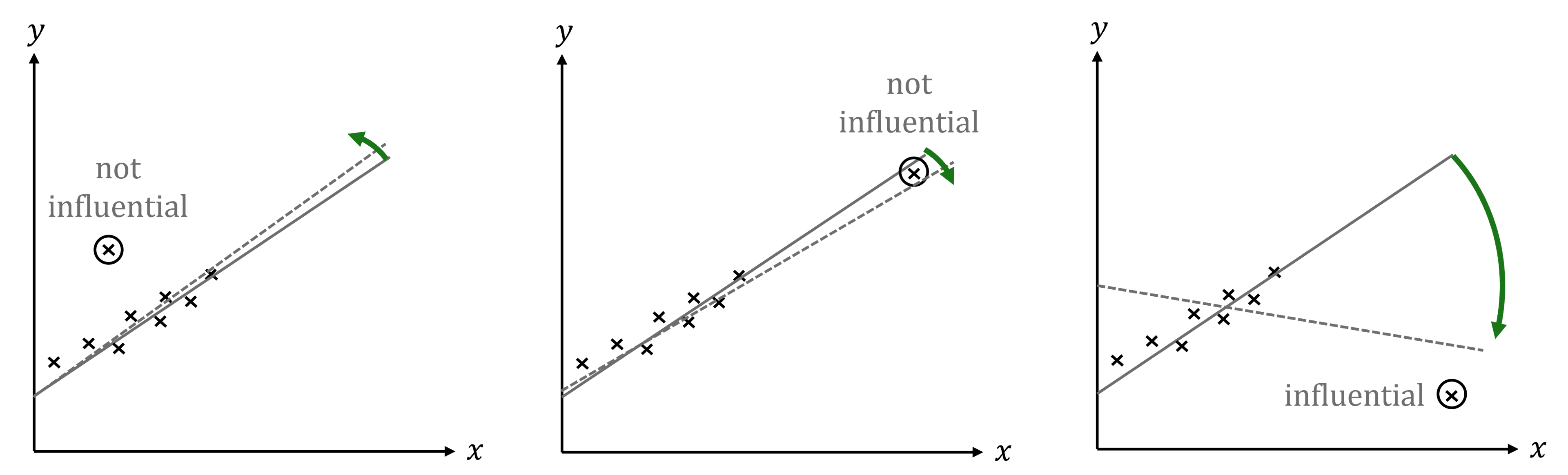
You've read 0 of your 10 free revision notes
Unlock more, it's free!
Did this page help you?