The Least-Squares Regression Line (College Board AP® Statistics): Study Guide
Least-squares regression line
What is the least-squares regression line?
The least-squares regression line is a special type of regression line that:
minimizes the sum of the squares of the residuals
and that passes through the mean point
where
is the mean of the
-values
and
is the mean of the
-values
It is used to predict
-values from given
-values
Its full name is the least-squares regression line of
on
This is not the same line if you wanted to predict
-values from given
-values
That is the least-squares regression line of
on
You cannot swap
and
What is the sum of the squares of the residuals?
The sum of the squares of the residuals for any regression line is found by
calculating the residuals for each data point
squaring each residual
then adding together all these squared values
Why is the sum of the squares of the residuals minimized?
Residuals are like errors when comparing regression lines
A good regression line should minimize the residuals
So compare the sum of all the residuals for different regression lines
However, the sum of all the residuals is zero
The positive residuals end up cancelling out the negative ones
So, instead, compare the sum of the squares of the residuals
because squaring the residuals makes them all positive
which stops any cancellation
The regression line with the smallest possible sum of the squares of the residuals is the least-squares regression line
What is the equation of the least-squares regression line?
The equation of the least-squares regression line is given by
is the
-intercept
is the slope
note the order of the terms
where
is the
-value predicted by the regression line
This is usually different to the actual
-value of a data point
is the explanatory variable
where
is the correlation coefficient
and
which rearranges to
(to find
)
You need to find
before you can find
In practice, the equation of the least-squares regression line is found using technology
e.g. a calculator
Examiner Tips and Tricks
The formulas for the equation of the least-squares regression line are given in the exam.
How do I interpret the slope of a regression line?
The slope,
, of the regression line
is
the amount by which the predicted
-variable,
, changes for every 1 unit of increase in the
-variable
i.e. the increase in
per unit increase in
How do I interpret the y-intercept of a regression line?
The
-intercept,
, of the regression line
is
the predicted value of
when the explanatory variable,
, equals zero
In some contexts, the y-intercept may not have a logical interpretation
Worked Example
The scatterplot below shows the number of hours spent studying (on the -axis) against the score in a test out of 16 points (on the
-axis), for five different students.
The equations of three different regression lines are shown, together with sums of squares of their residuals in the table below. The variable is the predicted value of
. One of these three regression lines is the least-squares regression line.
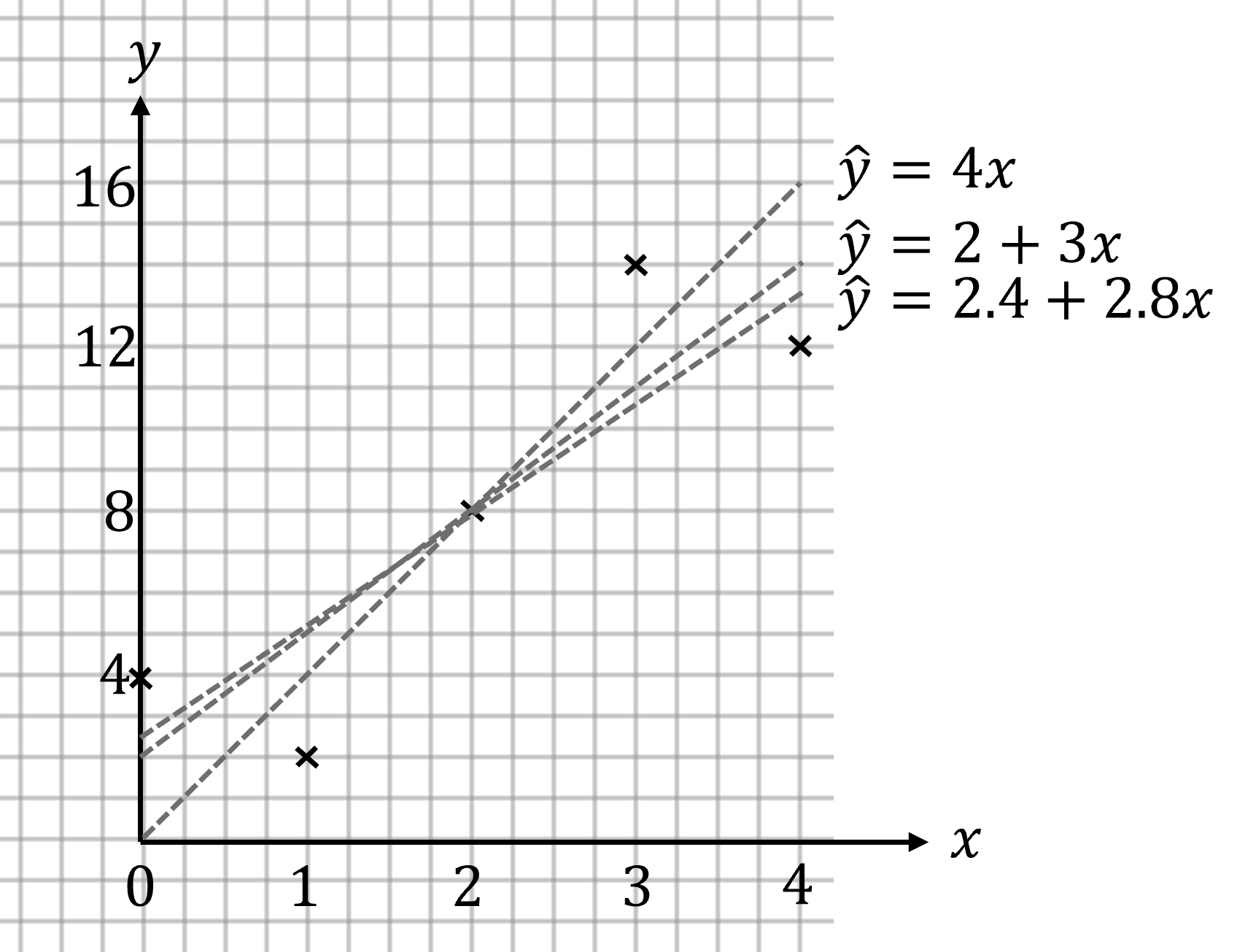
Regression equation | Sum of the squares of the residuals |
---|---|
25.6 | |
26 | |
40 |
(a) Explain how you know which regression line is the least-squares regression line.
Answer:
The least-squares regression line minimizes the sum of the squares of the residuals
The regression line has the smallest sum of the squares of the residuals, as 25.6 < 26 < 40
We are told that one of the three regression lines is the least-squares regression line
This means is the least-squares regression line
(b) Explain what the -intercept and the slope of the least-squares regression line mean in context.
Answer:
The -intercept of a regression line is the predicted value of
when
is zero
The slope of a regression line is the amount of change in the predicted value of for every increase by 1 in the value of
The -intercept shows that a student who has done no studying is predicted to score 2.4 (which rounds to 2 points) out of 16
The slope shows that the predicted score of a student increases by 2.8 points per hour of studying
You've read 0 of your 5 free study guides this week
Unlock more, it's free!
Did this page help you?